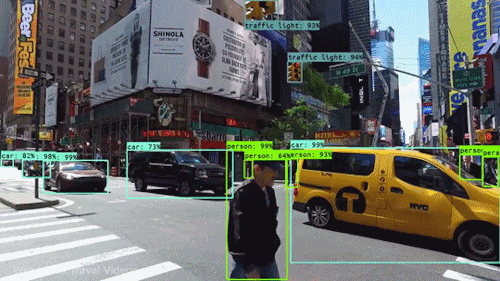
The Visionlab is a leader in innovative research within the field of computer vision. Directed by Luigi Cinque, our lab develops groundbreaking technologies and methodologies that significantly advance how computers interpret visual data, enhancing machine-human interactions and driving the evolution of autonomous systems.
Research Areas:
- Image and Video Analysis:
Our laboratory specializes in the development of state-of-the-art techniques for image segmentation, motion detection, and visual saliency. These capabilities are crucial for applications ranging from environmental monitoring to advanced video editing and content curation tools. - Machine/Deep Learning for Vision:
We employ advanced statistical models and neural networks to teach machines how to interpret and understand visual information. Our work includes the development of novel training methods that reduce the need for labeled data and the exploration of generative models to create high-fidelity visual simulations for training purposes. - Human-Computer Interaction:
We are pioneering new methods for real-time multi-modal interaction, integrating visual data with audio and tactile feedback to create more immersive and intuitive user experiences. Our research includes the development of advanced virtual assistants that use visual cues to understand user needs better. - Autonomous Systems:
Our laboratory is at the forefront of developing computer vision technologies for autonomous robotics in complex and unstructured environments. This includes deep reinforcement learning models that enable robots to make decisions based on visual inputs and advanced perception systems that can adapt to various lighting and weather conditions. - Medical Imaging and Healthcare Applications:
We explore the application of computer vision in medical imaging, including diagnosis assistance, surgical guidance, and patient monitoring. Our research aims to improve the accuracy and efficiency of medical procedures while reducing healthcare costs and improving patient outcomes. - Visual Scene Understanding:
Our laboratory is dedicated to advancing the understanding of visual scenes, including object detection, scene segmentation, and contextual understanding. This research is fundamental for various applications such as autonomous navigation, augmented reality, and urban planning. - Cross-Modal Fusion:
We investigate methods for integrating information from multiple sensory modalities, such as vision, audio, and text, to improve understanding and decision-making capabilities. This research has applications in multimedia analysis, assistive technologies, and human-robot interaction. - Privacy-Preserving Computer Vision:
In response to growing concerns about privacy and data security, our lab develops techniques for performing computer vision tasks while preserving the privacy of individuals’ visual data. This includes methods for anonymization, secure computation, and decentralized processing.
Explore our pubblications